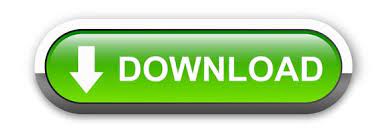
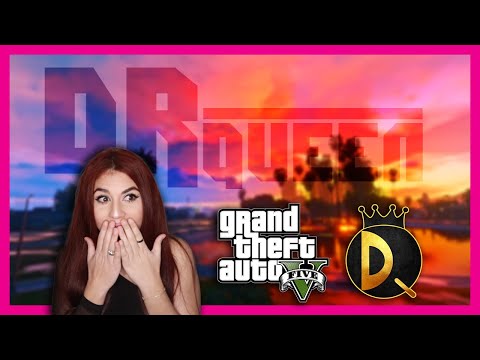
MSE-efficient GMM estimator involves a weighting matrix different from the Using theįramework of GMM with local misspecification as proposed by Newey (1985), weĪre able to devise a GMM estimator with minimum asymptotic MSE. Their likely endogeneity, because they are seen as highly relevant. Underpin the common practice of using some instrumental variables, in spite of Work in ProgressĪbstract: This paper provides a new asymptotic theory to Implies testing for non-nested hypotheses. We argue that a proper assessment of Granger causality Sims and Granger concepts of non-causality and the Log-Likelihood Ratio testsįor each of them. We set a specialįocus on the design of measures that properly disentangle these two components.įurthermore, this disentangling leads us to revisit the equivalence between the The amount of causality because what they consider as a causality measure mayĪlso convey a measure of the amount of (non)-markovianity. Relevant conditioning information? In this paper we argue that this common featureīetween (non)-markovianity and Granger causality has led people to overestimate Prediction: how much is the forecast accuracy deteriorated when forgetting some To set the focus on the interplay between measurement of (non)-markovianity and (1982) work in the context of Gaussian VAR processes, Schreiber (2000) has set a special focus on GrangerĬausality and dubbed the same measure “transfer entropy.” Both papers measureĬausality in the context of Markov processes. Had introduced the so-called “Kullback Causality Measures”, extending Geweke’s Proposed for a quantitative assessment of causality relationships. Carter Hill editors, Emerald Group Publishing.)Ībstract: Many Information Theoretic Measures have been “ Causality and Markovianity: Information Theoretic Measures” (with Eric Renault – revise & resubmit in: “Advances in Econometrics: Essays in Honor of Aman Ullah”, Vol 36, Gloria Gonzalez-Rivera, Tae-Hwy Lee, and R. After theĬrisis, the network influence decreased, though it did not return to pre-crisis Network strength was the highest during the financial crisis in 2008. Measures and graphical tools to interpret the network effects. Stock index volatility data in the 2003-2015 period. The methodology is illustrated through anĪpplication to financial integration among countries based on daily realized

Restrictiveness of the constraints imposed by the spatial model on the SVAR One possible identification strategy of SVAR models.
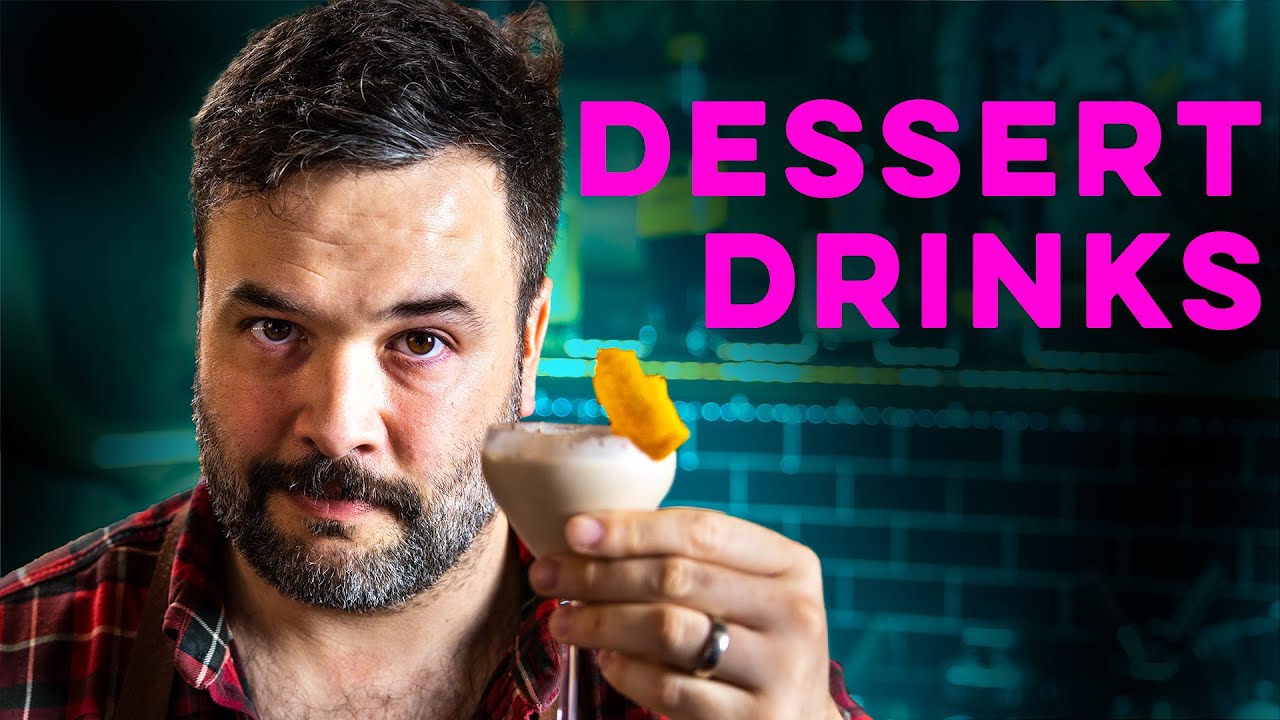
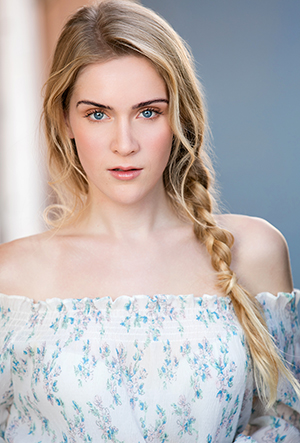
I discuss machine learning methods, based on the PC-algorithm, as (row-standardized) network matrix and the strength parameter from the SVARĮstimates. This paper is to propose a two-step minimum distance approach to estimate the Based on these restrictions, the main theoretical contribution of
DANIELA SCIDA BROWN SERIES
I show thatĪ time series spatial model is a constrained Structural Vector Autoregressive Network matrix and the network strength in a time series framework. In this paper, I put forth a methodology to estimate both the Ties are seldom available, researchers have mostly relied on ad-hoc network Of the overall strength of the network ties and require the researcher to knowĪ-priori how individuals are connected to each other. However, these models only provide an estimate I am originally from Tucumán, a small province in the north of Argentina.Abstract: Spatial models are a natural way to analyze I received my undergraduate degree in Economics at Universidad Nacional de Tucumán in Argentina, and my masters degree in Economics and Finance at CEMFI in Madrid, Spain. I also developed a pre-college course in econometrics for summer school at Brown at an AP Statistics level. Until recently, I was also the co-coordinator of our QSR Seminars and (virtual) Brown Bags series.ĭuring my time at Brown University, I was a teaching assistant for financial econometrics among other econometric courses, at both graduate and undergraduate levels. My supervisory experience includes model development and forecasting under stress conditions, as well as examination from participation in the CCAR program. I was part of the Federal Reserve System stress testing program until June 2022. On the supervisory side, I currently lead an innovation project that leverages NLP methods and mapping techniques. Many of my current research projects focus on financial networks estimation and, more recently, at the intersection with NLP methods. I am particularly interested in applied econometrics and econometric methodology for time series. My research interests lie in the areas of time series analysis, financial econometrics, and financial networks. Before joining the Richmond Fed in 2016, I completed my Ph.D. I am a M anager Financial Economist in the Quantitative Supervision & Research (QSR) group of the Federal Reserve Bank of Richmond.
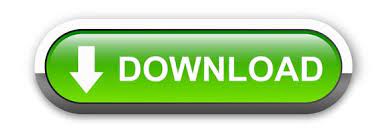